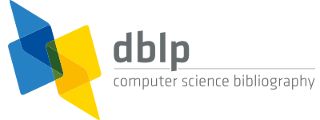


default search action
2nd MILLanD@MICCAI 2023: Vancouver, BC, Canada
- Zhiyun Xue
, Sameer K. Antani
, Ghada Zamzmi
, Feng Yang
, Sivaramakrishnan Rajaraman
, Sharon Xiaolei Huang
, Marius George Linguraru
, Zhaohui Liang
:
Medical Image Learning with Limited and Noisy Data - Second International Workshop, MILLanD 2023, Held in Conjunction with MICCAI 2023, Vancouver, BC, Canada, October 8, 2023, Proceedings. Lecture Notes in Computer Science 14307, Springer 2023, ISBN 978-3-031-47196-4
Efficient Annotation and Training Strategies
- Serban Vadineanu
, Daniël Maria Pelt, Oleh Dzyubachyk, Kees Joost Batenburg:
Reducing Manual Annotation Costs for Cell Segmentation by Upgrading Low-Quality Annotations. 3-13 - Yijie Qu, Qianfei Zhao, Linda Wei, Tao Lu, Shaoting Zhang, Guotai Wang:
ScribSD: Scribble-Supervised Fetal MRI Segmentation Based on Simultaneous Feature and Prediction Self-distillation. 14-23 - Nazanin Moradinasab
, Rebecca A. Deaton
, Laura S. Shankman
, Gary K. Owens
, Donald E. Brown
:
Label-Efficient Contrastive Learning-Based Model for Nuclei Detection and Classification in 3D Cardiovascular Immunofluorescent Images. 24-34 - Christopher Adnel, Islem Rekik
:
Affordable Graph Neural Network Framework Using Topological Graph Contraction. 35-46
Approaches for Noisy, Missing, and Low Quality Data
- Xiongchao Chen, Bo Zhou, Huidong Xie
, Xueqi Guo, Qiong Liu, Albert J. Sinusas, Chi Liu:
Dual-Domain Iterative Network with Adaptive Data Consistency for Joint Denoising and Few-Angle Reconstruction of Low-Dose Cardiac SPECT. 49-59 - Yang Yu
, Jiahao Wang, Ashish Jith Sreejith Kumar, Bryan Tan, Navya Vanjavaka, Nurul Hafidzah Rahim, Alistair Koh, Shaheen Low, Yih Yian Sitoh, Hanry Yu, Pavitra Krishnaswamy, Ivan Ho Mien:
A Multitask Framework for Label Refinement and Lesion Segmentation in Clinical Brain Imaging. 60-70 - Maryna Kvasnytsia
, Abel Díaz Berenguer
, Hichem Sahli
, Jef Vandemeulebroucke
:
COVID-19 Lesion Segmentation Framework for the Contrast-Enhanced CT in the Absence of Contrast-Enhanced CT Annotations. 71-81 - Can Cui, Yaohong Wang, Shunxing Bao, Yucheng Tang, Ruining Deng, Lucas W. Remedios, Zuhayr Asad, Joseph T. Roland, Ken S. Lau, Qi Liu, Lori A. Coburn, Keith T. Wilson
, Bennett A. Landman, Yuankai Huo:
Feasibility of Universal Anomaly Detection Without Knowing the Abnormality in Medical Images. 82-92
Unsupervised, Self-supervised, and Contrastive Learning
- Camille Ruppli, Pietro Gori, Roberto Ardon, Isabelle Bloch:
Decoupled Conditional Contrastive Learning with Variable Metadata for Prostate Lesion Detection. 95-105 - Yunsung Chung, Chanho Lim, Chao Huang, Nassir Marrouche, Jihun Hamm:
FBA-Net: Foreground and Background Aware Contrastive Learning for Semi-Supervised Atrium Segmentation. 106-116 - Tony Xu
, Matthew Rozak
, Emmanuel E. Ntiri, Adrienne Dorr
, James R. Mester, Bojana Stefanovic, Anne L. Martel
, Maged Goubran
:
Masked Image Modeling for Label-Efficient Segmentation in Two-Photon Excitation Microscopy. 117-127 - Zhaohui Liang
, Zhiyun Xue
, Sivaramakrishnan Rajaraman
, Feng Yang
, Sameer K. Antani
:
Automatic Quantification of COVID-19 Pulmonary Edema by Self-supervised Contrastive Learning. 128-137 - Rahul G. S., Sriprabha Ramanarayanan, Mohammad Al Fahim
, Keerthi Ram, Preejith S. P, Mohanasankar Sivaprakasam:
SDLFormer: A Sparse and Dense Locality-Enhanced Transformer for Accelerated MR Image Reconstruction. 138-147 - Slim Hachicha, Célia Le, Valentine Wargnier-Dauchelle, Michaël Sdika:
Robust Unsupervised Image to Template Registration Without Image Similarity Loss. 148-157 - Jianfei Liu, Omid Shafaat, Ronald M. Summers:
A Dual-Branch Network with Mixed and Self-Supervision for Medical Image Segmentation: An Application to Segment Edematous Adipose Tissue. 158-167
Weakly-Supervised, Semi-supervised, and Multitask Learning
- Sajith Rajapaksa, Khashayar Namdar, Farzad Khalvati:
Combining Weakly Supervised Segmentation with Multitask Learning for Improved 3D MRI Brain Tumour Classification. 171-180 - Ziyang Wang, Irina Voiculescu:
Exigent Examiner and Mean Teacher: An Advanced 3D CNN-Based Semi-Supervised Brain Tumor Segmentation Framework. 181-190 - Peidi Xu
, Blaire Lee, Olga V. Sosnovtseva, Charlotte Mehlin Sørensen
, Kenny Erleben
, Sune Darkner:
Extremely Weakly-Supervised Blood Vessel Segmentation with Physiologically Based Synthesis and Domain Adaptation. 191-201 - Krishna Thoriya, Preeti Mutreja, Sumit Kalra, Angshuman Paul:
Multi-task Learning for Few-Shot Differential Diagnosis of Breast Cancer Histopathology Images. 202-210
Active Learning
- Bella Specktor-Fadida
, Anna Levchakov, Dana Schonberger, Liat Ben-Sira, Dafna Ben-Bashat, Leo Joskowicz
:
Test-Time Augmentation-Based Active Learning and Self-training for Label-Efficient Segmentation. 213-223 - Ji Wu, Zhongfeng Kang, Sebastian Nørgaard Llambias, Mostafa Mehdipour-Ghazi
, Mads Nielsen:
Active Transfer Learning for 3D Hippocampus Segmentation. 224-234
Transfer Learning
- Gino Gulamhussene
, Oleksii Bashkanov, Jazan Omari, Maciej Pech
, Christian Hansen
, Marko Rak:
Using Training Samples as Transitive Information Bridges in Predicted 4D MRI. 237-245 - Tushar Kataria, Beatrice Knudsen, Shireen Y. Elhabian:
To Pretrain or Not to Pretrain? A Case Study of Domain-Specific Pretraining for Semantic Segmentation in Histopathology. 246-256 - Masakata Kawai
, Noriaki Ota, Shinsuke Yamaoka:
Large-Scale Pretraining on Pathological Images for Fine-Tuning of Small Pathological Benchmarks. 257-267

manage site settings
To protect your privacy, all features that rely on external API calls from your browser are turned off by default. You need to opt-in for them to become active. All settings here will be stored as cookies with your web browser. For more information see our F.A.Q.