News & Articles
Using AI to help healthcare providers in the cancer space
Color

We believe there are opportunities to improve healthcare provider satisfaction and efficiency, as well as patient outcomes, by using AI to create tools for providers and their teams.
The current challenges of cancer care in the United States mirror many of the challenges seen across the broader healthcare landscape:
- Providers are overworked.
- Patients struggle to afford screenings and treatment.
- Patients do not know which screenings they should be doing (or they aren’t incentivized to do them, given all other obstacles in healthcare and life).
- When something abnormal comes up, the system is fragmented and difficult to navigate.
- Many who are diagnosed with cancer are left to figure out what to do next, right at a time where the rug has been pulled out from underneath them emotionally.
The Problem: Primary Care’s Challenges in Evaluating Cancer Risk
Several years ago, I (Keegan) was working as a primary care physician when I cared for a woman in her 30s whose aunt had just been diagnosed with pancreatic cancer. The woman had been close to her aunt while growing up, so the news profoundly affected her. She was concerned not only for her aunt but also for her own cancer risk. As we delved into her family history, we discovered that her uncle on the same side of the family had developed liver cancer and passed away in his 70s. It was believed the cancer had metastasized from another origin, possibly the prostate, which further obscured my patient’s true risk.
This was a complex case. I began to wonder about my patient’s hereditary risk for cancer and the implications for her growing family—she had two young children. I suspected there were specific testing and screening guidelines tailored to her situation, but being fresh out of residency, I was uncertain about next steps. Although I knew the NCCN (National Comprehensive Cancer Network) had guidelines for screening individuals at increased risk for cancer, I found them dense and challenging to understand. I questioned my ability to correctly interpret them. How would I ensure I was using the most up-to-date information? What if I recommended an evaluation or test that insurance wouldn’t cover? Was I confident enough in my recommendation that she would not have to pay out of pocket for screening?
As primary care physicians, we are often expected to address numerous conditions within a brief 15-minute visit. While annual physicals offer an opportunity to discuss cancer screening, they are also consumed by managing complex conditions like diabetes, asthma, and depression. Consequently, cancer risk assessment and screening can sometimes be sidelined, especially in patients with a complicated family history indicating a hidden risk.
In the end, I decided it was safer to refer my patient to a high risk cancer clinic rather than handle the decision-making myself. The time required to research the guidelines, gather a comprehensive family and medical history, and develop a screening plan was more than I had available in the middle of a busy clinic. It was undoubtedly not the ideal experience for my patient. Instead of having a clear plan before leaving the office that day, she had to wait several weeks for a specialist appointment, prolonging her anxiety.
If the guidelines were easier to interpret or I had a tool to input her risk factors and receive the appropriate guidelines-based screening or testing recommendations, I could have developed a plan for her on the spot, sparing her the cost of a specialist follow-up and the stress of uncertainty. This would’ve empowered me to practice at the top of my license and fulfill a critical role of the primary care provider—assessing cancer risk and providing meaningful, guidelines-based recommendations for screening.
How AI can help
Large Language Models (LLMs) have super powers in helping with three key areas:
Extracting meaning from a patient’s medical records
Whether it is to determine the most appropriate cancer screening plan for a patient or to identify if there are any workup gaps before creating a cancer treatment plan, healthcare providers and their teams spend hours reviewing a patient’s prior test results, visit notes, and health history information.

This is where LLMs come in – they are able to analyze large data sets, including unstructured health data, using natural language processing (NLP). With an LLM it currently takes seconds to review a patient’s medical records and extract pertinent information. These records include multiple visit notes in various formats; not just structured information stored in discrete fields in an electronic health record (EHR).
Tip: If you are working with an external AI company, make sure they are HIPAA compliant and that you have proper BAAs in place. A patient’s medical information should not be used as training data for an LLM.
Finding patient-relevant details within complicated guidelines and papers
The other side of the puzzle for providers is staying on top of regularly changing guidelines and new evidence from clinical trials. We are fortunate that there are advances in early cancer detection and treatment, but it can make it very challenging for providers to stay on top of everything.
For those doctors that do have the time, or who have specialized training in areas like genetic counseling and medical oncology, there are widely trusted sources they regularly use to guide care for their patients. Some examples are the NCCN, the United States Preventive Services Task Force (USPSTF), the American College of Obstetricians and Gynecologists (ACOG), UpToDate, the Journal of Clinical Oncology, the Lancet, and trusted colleagues.

LLMs are skilled at parsing out these often complicated, lengthy medical guidelines and studies to pull out up-to-date, relevant information.
Tip: One challenge for LLMs is that they tend to not contain the most recent information. It is important that your strategy considers how to proactively check for recent updates in medical guidelines and the like, as well as how to handle disagreement between sources.
Connecting the dots between patient info and guidelines
Once you have thorough knowledge of both patient-specific details and relevant guidelines, doctors then need to make the right decisions on how they connect in context of a specific task. For example, it can be challenging for the average primary care provider (PCP) to know if a patient with unusual family history should be screened for colorectal cancer earlier than what is considered standard of care, or if they should have a breast MRI in addition to a mammogram to screen for breast cancer. Even more time and work gets layered on when you consider documentation needed by insurance companies for related imaging, labs and procedures.
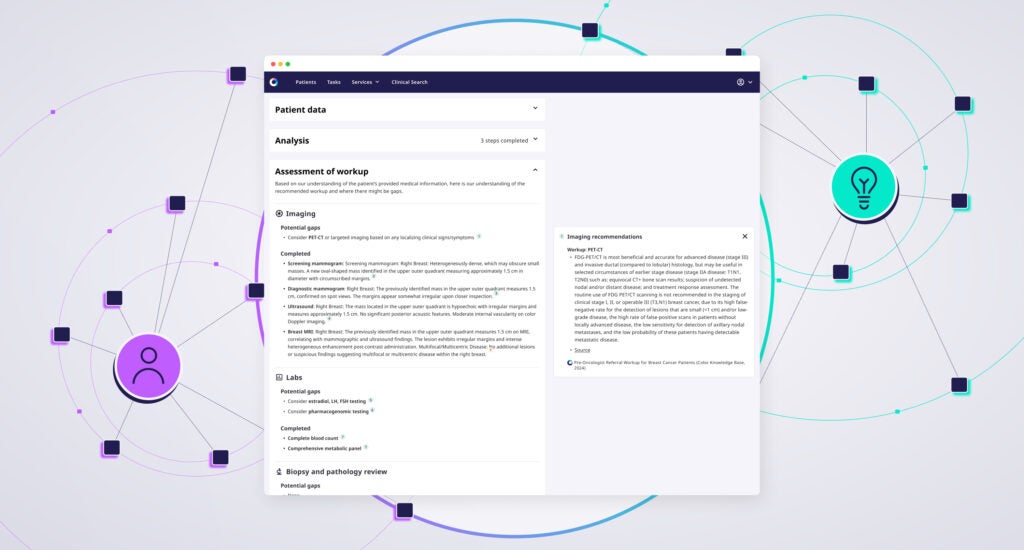
LLMs use deep neural networks to process text and generate responses based on learned patterns. Their ability to answer specific questions (“what is the right breast cancer screening plan”) against patient specific information (“for patient X”) and high-quality guidelines (“based on trusted breast cancer screening guidelines”) is an excellent example of this.
Additionally, LLMs’ ability to creatively generate content is useful when generating prior authorization documentation needed by insurance companies. Using information about the patient + provider + preferred clinical guidelines information, along with CPT and ICD-10 codes, prior authorization documentation can be automatically generated for review by clinical teams.
AI augments clinical decision making; it doesn’t replace it
The LLM “superpowers” mentioned above are all in support of the jobs healthcare providers (and their teams) already do today. Our intent is to create tools for them to do their work more efficiently, and potentially with a broader scope, so that they can spend more time with their patients. While AI may help us improve our effectiveness, there is an art to medicine that it can’t fully stand in for. It is very important that providers carefully review the output of these models and have a hand in developing them. In AI circles, systems like this are commonly referred to as “Human in the Loop” systems. The end user is an individual who has the skills to assess the quality of the system and decide how to use it.
Tip: It is well-established in clinician circles that user experience improvements are needed for most healthcare software tools. Software teams should strive to create tools that are easy to use and embedded in software healthcare providers are already using.
Whether it’s extracting meaningful data from medical records, parsing complicated guidelines, or finding the appropriate screening or workup plan for a patient, it’s clear that AI has the potential to be a powerful ally in the clinical setting. These tools can empower primary care providers and other clinicians to provide the most up-to-date, evidence-based cancer care with a degree of confidence and speed that was, until recently, just out of reach. By responsibly integrating AI tools within the context of real-world needs, we aim to support clinicians in patient care, enhancing their efficiency and broadening their scope of practice, while ultimately improving patient outcomes. At Color, we are committed to developing solutions that not only address these challenges but also ensure that the human touch remains at the heart of medical care.
To learn more, go to color.com/copilot or reach out at copilot@color.com